Rémi Emonet's Research Home (see global home)
Selection of publications (see all publications and details)
- Year 2022COptimal Tensor Transport2022- AAAI (AAAI Conference on Artificial Intelligence)[gs][bibtex] (click to show)
@inproceedings{kerdoncuff2022optimal, title={Optimal Tensor Transport}, author={Kerdoncuff, Tanguy and Perrot, Micha{\"e}l and Emonet, R{\'e}mi and Sebban, Marc}, booktitle={Proceedings (AAAI Artificial Intelligence Conference)}, year={2022} }
pub87 - Year 2021CLearning Stochastic Majority Votes by Minimizing a PAC-Bayes Generalization Bound2021- NeurIPS (Advances in Neural Information Processing Systems)[gs][bibtex] (click to show)
@article{zantedeschi2021learning, title={Learning Stochastic Majority Votes by Minimizing a PAC-Bayes Generalization Bound}, author={Zantedeschi, Valentina and Viallard, Paul and Morvant, Emilie and Emonet, R{\'e}mi and Habrard, Amaury and Germain, Pascal and Guedj, Benjamin}, journal={Advances in Neural Information Processing Systems}, volume={34}, year={2021} }
pub84 - Year 2019CAn Adjusted Nearest Neighbor Algorithm Maximizing the F-measure from Imbalanced Data2019- ICTAI (International Conference on Tools with Artificial Intelligence)[gs][bibtex] (click to show)
@inproceedings{metzler2019gnn, title={An Adjusted Nearest Neighbor Algorithm Maximizing the F-measure from Imbalanced Data}, author={ and Emonet, R{\'e}mi and Fromont, Elisa}, booktitle={IEEE International Conference on Image Processing}, year={2019} }
pub071CFrom Cost-Sensitive to Tight F-measure Bounds2019- AISTATS (International Conference on Artificial Intelligence and Statistics)[gs][bibtex] (click to show)@inproceedings{bascolmetzlerfmeasure, title={From Cost-Sensitive to Tight F-measure Bounds}, author={Bascol, Kevin and Emonet, Rémi and Fromont, Elisa and Habrard, Amaury and Metzler, Guillaume and Sebban, Marc}, booktitle={AISTATS 2019}, year={2019}, comment={CONE}, }
pub063 - Year 2017CResidual Conv-Deconv Grid Network for Semantic Segmentation2017- BMVC (British Machine Vision Conference)https://github.com/Fourure/GridNet[gs][bibtex] (click to show)
@inproceedings{fourure2017gridnet, title={Residual Conv-Deconv Grid Network for Semantic Segmentation}, author={Fourure, Damien and Emonet, R{\'e}mi and Fromont, Elisa and Muselet, Damien and Tr{\'e}meau, Alain and Wolf, Christian}, booktitle={Proceedings of the British Machine Vision Conference, 2017}, year={2017} }
pub053JMulti-task, Multi-domain Learning: application to semantic segmentation and pose regression2017- Neurocomputing[gs][bibtex] (click to show)@article{fourure2017multi, title={Multi-task, Multi-domain Learning: application to semantic segmentation and pose regression}, author={Fourure, Damien and Emonet, R{\'e}mi and Fromont, Elisa and Muselet, Damien and Neverova, Natalia and Tr{\'e}meau, Alain and Wolf, Christian}, journal={Neurocomputing}, volume={251}, pages={68--80}, year={2017}, publisher={Elsevier} }
pub052 - Year 2015CLandmarks-based Kernelized Subspace Alignment for Unsupervised Domain Adaptation2015- CVPR (IEEE conf. Computer Vision and Pattern Recognition)[gs][bibtex] (click to show)
@inproceedings{aljundi2015landmarks, title={Landmarks-based Kernelized Subspace Alignment for Unsupervised Domain Adaptation}, author={Aljundi, Rahaf and Emonet, R{\'e}mi and Muselet, Damien and Sebban, Marc}, booktitle={Computer Vision and Pattern Recognition}, year={2015} }
pub041 - Year 2014JTemporal Analysis of Motif Mixtures using Dirichlet Processes2014- TPAMI (Transactions on Pattern Analysis and Machine Intelligence)[gs][bibtex] (click to show)
@ARTICLE{Emonet_PAMI_2014, author = {Emonet, Remi and Varadarajan, Jagannadan and Odobez, Jean-Marc}, title = {Temporal Analysis of Motif Mixtures using Dirichlet Processes}, journal = {IEEE Transactions on Pattern Analysis and Machine Intelligence}, year = {2014} }
pub030 - Year -[gs]-[gs]-[gs]-[gs]-[gs]-[gs]-[gs]-[gs]-[gs]-[gs]-[gs]-[gs]-[gs]-[gs]-[gs]-[gs]-[gs]-[gs]-[gs]-[gs]-[gs]-[gs]-[gs]-[gs]-[gs]-[gs]-[gs]-[gs]-[gs]-[gs]-[gs]-[gs]-[gs]-[gs]-[gs]-[gs]-[gs]-[gs]-[gs]-[gs]-[gs]-[gs]-[gs]-[gs]-[gs]-[gs]-[gs]-[gs]-[gs]-[gs]-[gs]-[gs]-[gs]-[gs]-[gs]
- [C]Optimal Tensor Transport
2022 − Core A* − AAAI (AAAI Conference on Artificial Intelligence) - [C]Learning Stochastic Majority Votes by Minimizing a PAC-Bayes Generalization Bound
2021 − Core A* − NeurIPS (Advances in Neural Information Processing Systems) - [C]An Adjusted Nearest Neighbor Algorithm Maximizing the F-measure from Imbalanced Data
2019 − Core B − ICTAI (International Conference on Tools with Artificial Intelligence) - [C]From Cost-Sensitive to Tight F-measure Bounds
2019 − Core A − AISTATS (International Conference on Artificial Intelligence and Statistics) - [C]Residual Conv-Deconv Grid Network for Semantic Segmentation
2017 − Core B − BMVC (British Machine Vision Conference) - [J]Multi-task, Multi-domain Learning: application to semantic segmentation and pose regression
2017 − Q1 − IF 3.317 − Neurocomputing - [C]Landmarks-based Kernelized Subspace Alignment for Unsupervised Domain Adaptation
2015 − Core A − CVPR (IEEE conf. Computer Vision and Pattern Recognition) - [J]Temporal Analysis of Motif Mixtures using Dirichlet Processes
2014 − Q1 − IF 5.694 − TPAMI (Transactions on Pattern Analysis and Machine Intelligence)
Demos
For now, visit my videos page.
Presentations (research and related)
Here are presentations that I made publicly enough so that it makes sense to share them. (click the icon on the left to see the sources and a PDF export)
[2022-06-16] Unsupervised Decomposition of Images into Motifs (GDR Appamat)
[2021-11-18] Deep Equilibrium Models and Implicit Layers (LabHC)
[2021-11-16] Optimal Transport Based RNN Distillation (ANR TAUDoS)
[2021-07-06] Learning from Imbalanced Data and Anomaly Detection (EUR Sleight SSE6)


[2019-06-27] Behavior of Distance-Based Methods (LACODAM Team)

[2018-06-07] A Tour of Machine Learning and its Subdomains (LabHC)














[2014-03-10] Temporal Topic Models for Probabilistic Motif Mining − SMiLe2014


Research Software and Related Projects
See also individual links in my publication list, or a list of paper-related github repositories.


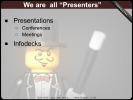


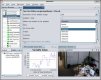